Choosing between artificial intelligence and generative AI can be confusing. Traditional AI has been around for a while. This article will show you how each type is different and why it matters when it comes to Artificial Intelligence vs Generative AI.
Keep reading to find out more!
Defining AI and Generative AI
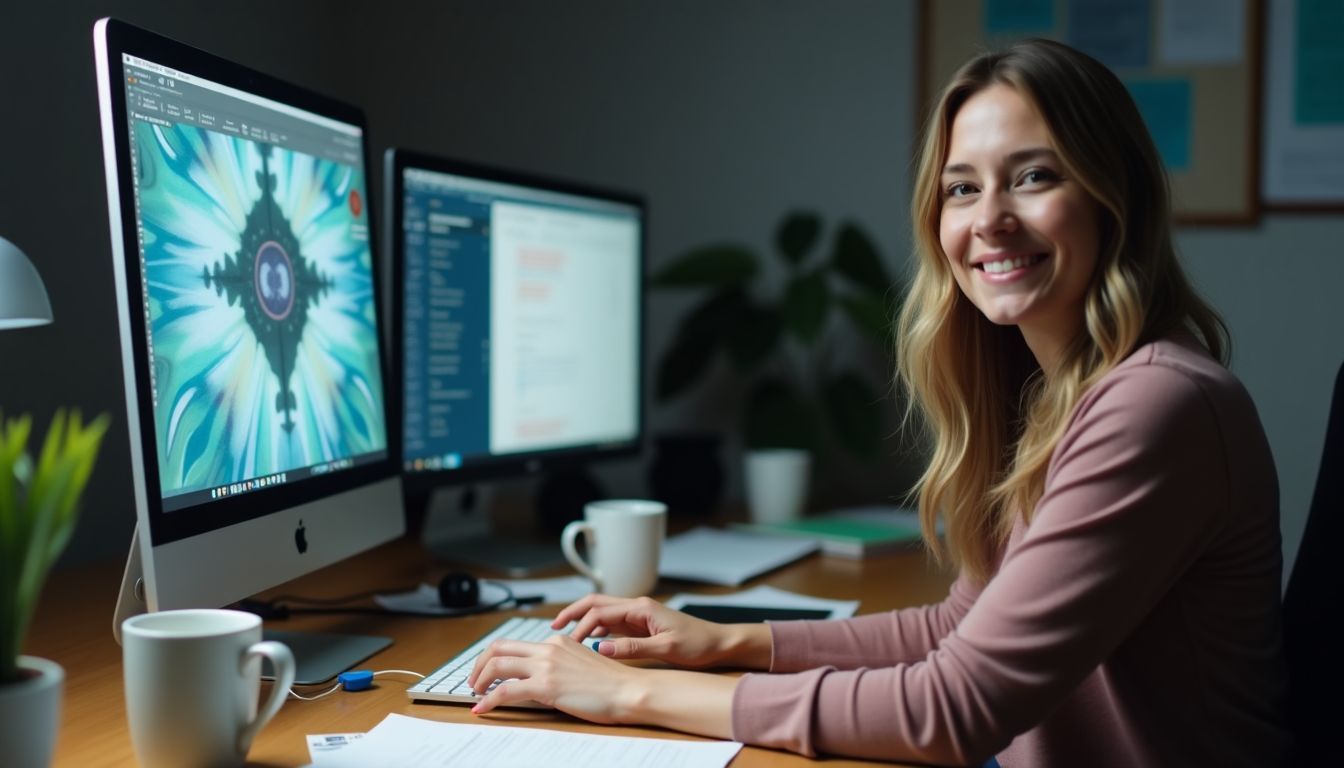
AI, or artificial intelligence, is the simulation of human intelligence processes by computer systems. Generative AI involves using machine learning to create new content or generate real-like outputs.
What is Traditional AI?
Traditional AI, often called Narrow or Weak AI, focuses on doing specific jobs well. It’s like giving a computer one task and it learns how to do it really well. Think of playing chess on a computer, asking Siri for the weather, or getting movie suggestions from Netflix.
This kind of AI looks at data and patterns to predict what might happen next.
Traditional AI powers many tools we use every day, from voice assistants to search engines.
This type includes things such as Google’s search tricks that help you find what you need fast or the smart systems behind Amazon’s recommendations. These AIs are programmed with certain rules to analyze information and make smart guesses based on what they’ve seen before.
But their learning is limited — they’re very good at their specific tasks but can’t do much outside of those boundaries.
What is Generative AI?
Generative AI is a part of artificial intelligence that makes new content. It takes in inputs and from them, makes original stuff. For example, GPT-4 by OpenAI is a type of generative AI.
It predicts what to write next based on what you give it. This kind of AI uses deep learning to work its magic. It learns from lots of data using something called neural networks.
One cool thing about generative AI is how it can make something out of nothing using prompts. It uses smart algorithms like GANs (Generative Adversarial Networks) and VAEs (Variational Autoencoders).
These tools help it create all sorts of things, from writing pieces to pictures. Generative AI has many uses because it’s good at coming up with new ideas from old ones.
Key Differences Between Traditional AI and Generative AI
Traditional AI focuses on solving specific tasks, while Generative AI is designed to create new content. The training and development process for Traditional AI involves supervised learning, whereas Generative AI often utilizes unsupervised or reinforcement learning techniques.
Focus and Output
Traditional AI operates on predefined rules and logic; Generative AI creates new content by understanding data patterns.
Conventional AI focuses on analyzing structured data and following logical reasoning to make decisions. This kind of AI excels at recognizing data patterns and giving probabilistic outputs based on rule-based systems.
It powers automated customer service by making use of structured data analysis.
Generative AI, on the other hand, shines in digital creativity. By working with both structured and unstructured data, it can generate innovative content such as digital art, music compositions, and unique design ideas.
Unlike traditional models that rely heavily on labelled data, generative models like GANs (Generative Adversarial Networks) produce realistic results even from unlabeled datasets.
Training and Development
Training and development in traditional AI involves educating the system using explicit rules. Machine learning algorithms are employed to teach the system to identify data patterns.
This educational process is typically grounded in a set of predefined rules and parameters, and it demands careful supervision by human trainers.
Conversely, generative AI employs deep learning techniques, specifically neural networks, for its training and development. It surpasses traditional AI as it can learn from vast amounts of data to generate new content that resembles human-created material without being explicitly programmed.
This approach enables generative AI systems to continuously progress and enhance through exposure to diverse datasets, contributing to their capacity to produce innovative outcomes.
User Interaction
Transitioning from the development process to user interaction, Generative AI utilizes adaptive learning and user input to improve engagement. It adjusts to new data, delivering personalized experiences by interpreting and integrating unique user feedback.
This adaptability allows for a more dynamic and customized approach towards user confidence and interpretability challenges. On the other hand, Traditional AI offers consistent results based on fixed inputs, limiting its capacity to dynamically personalize interactions or adjust based on user input.
This can lead to a less captivating experience compared to Generative AI’s interactive technology.
Generative AI’s focus on evolving through real-time data adaptation promotes more personalized and interactive technology experiences. In contrast, Traditional AI depends on predefined parameters, potentially resulting in limitations in adapting to individual user needs and preferences.
The implementation of Generative AI aims to unveil secrets that provide firsthand experience for users by integrating their inputs into the system’s continuously developing design.
Innovative Applications of AI
Innovative Applications of AI include using predictive analytics in business for making informed decisions and automating processes in manufacturing to improve efficiency. These applications leverage machine learning and data analysis to drive advancements in various industries.
Predictive Analytics in Business
Predictive analytics in business uses historical data to predict future outcomes and trends. It helps in areas like weather forecasting and financial analysis, enhancing decision-making by identifying patterns in the past data.
However, it has limitations such as data quality issues, ethical concerns, and a lack of interpretability in complex models.
Moving on to automation in manufacturing…
Automation in Manufacturing
After exploring the innovative applications of AI in predictive analytics for business, it is essential to acknowledge its significant impact on automation in manufacturing. AI technologies have redefined industrial automation, machine learning, and smart manufacturing processes.
Advanced robotics and cognitive automation systems powered by AI have revolutionized industrial automation, significantly improving efficiency, accuracy, and productivity within manufacturing processes through automated quality assurance and data-driven manufacturing techniques.
One notable example includes the use of autonomous systems equipped with AI-driven quality control solutions that promptly identify defects during product inspections. Moreover, predictive maintenance strategies utilize AI to forecast equipment failures, effectively reducing downtime in manufacturing operations.
The integration of AI technologies supports a new era of smart manufacturing, reshaping the industry’s landscape while enhancing competitiveness and innovation.
Innovative Applications of Generative AI
Generative AI is revolutionizing content creation in media, transforming the way visuals and text are produced. It has also sparked advances in design and simulation within engineering, offering new capabilities for creating and testing innovative solutions.
Content Creation in Media
Generative AI has revolutionized content creation in media, generating text, images, audio, and video for various applications. This technology, including ChatGPT, showcases a significant increase in global interest due to its ability to produce diverse forms of content.
However, challenges such as copyright infringement and data privacy issues remain pertinent concerns in this innovative application of generative AI. It holds potential across industries but is accompanied by worries about academic integrity and plagiarism.
Moving forward to the next section on “Design and Simulation in Engineering”.
Design and Simulation in Engineering
Generative AI is revolutionizing engineering design and simulation. By analyzing consumer preferences and engineering constraints, it creates innovative vehicle designs. Deep learning models use data from experiments and CFD simulations for design applications.
Furthermore, Generative AI can rapidly produce numerous prototypes, enabling engineers to explore a wide range of options for their projects.
Moving forward to “Conclusion”…
Conclusion
Generative AI is reshaping the artificial intelligence landscape. Unlike traditional AI, it surpasses performing predefined tasks by generating original content. This innovation opens new opportunities for industries like media, design, and engineering.
Grasping these distinctions is crucial for embracing the future of AI innovations in various fields. Both traditional and generative AI play crucial roles in shaping our ever-evolving technological world.
FAQs
1. What is the difference between artificial intelligence and generative AI?
Artificial intelligence (AI) refers to computer systems that can perform tasks usually requiring human intelligence. Generative AI is a specific type of AI that creates new content, like text or images, based on input data.
2. How do innovations in generative AI impact businesses?
Innovations in generative AI help businesses create unique marketing materials quickly. They also enhance customer interactions by personalizing responses and automating content creation processes.
3. What are some applications of artificial intelligence?
Applications of artificial intelligence include speech recognition, image analysis, and predictive analytics. These tools help companies improve efficiency and make better decisions based on data insights.
4. Can both types of AI work together effectively?
Yes, combining artificial intelligence with generative AI can lead to improved outcomes. For instance, traditional AI can analyze data while generative AI produces tailored content from those insights for various uses.