Finding new drugs is hard and takes a lot of time. AI has helped make over 150 small-molecule drugs. This article shows how AI is used in drug discovery to solve these problems. Keep reading to learn more!
The Role of AI in Modern Drug Discovery
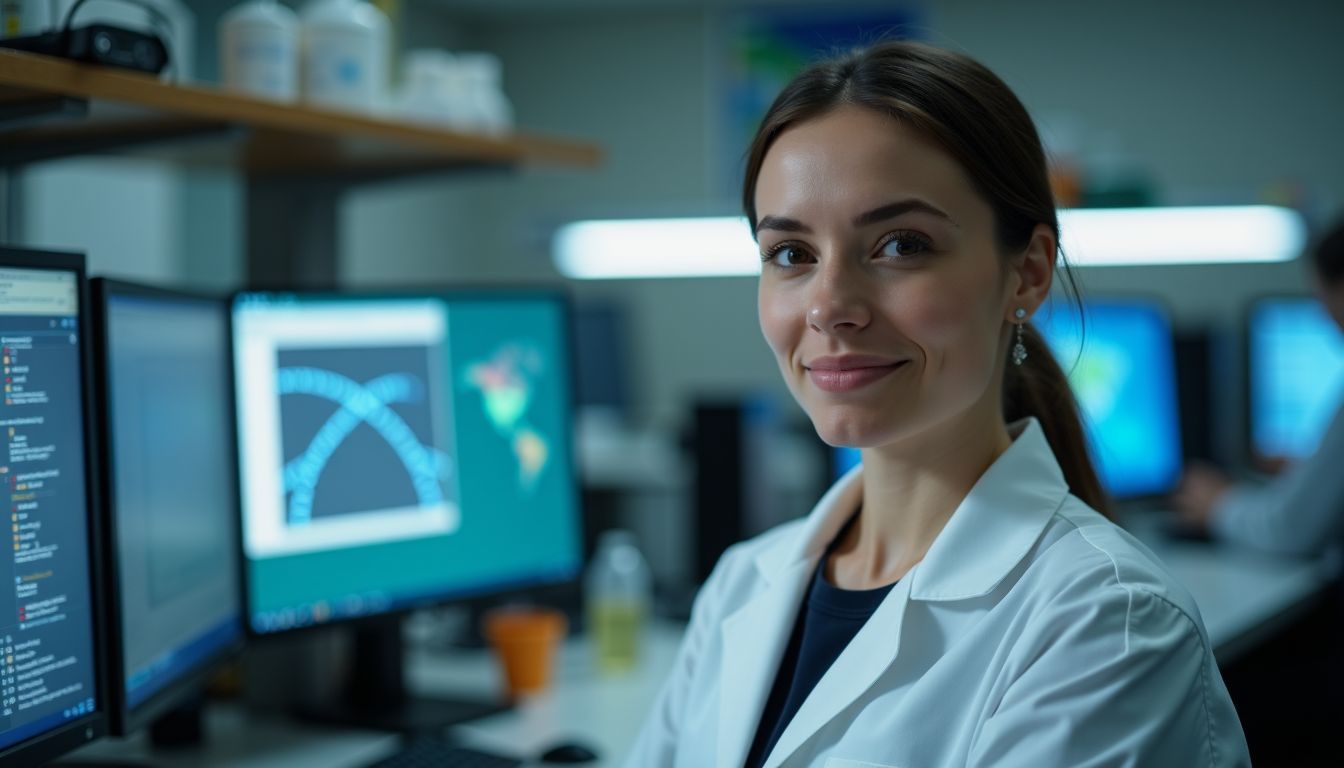
AI plays a crucial role in modern drug discovery by revolutionizing the way biopharmaceutical companies develop drugs. Machine learning models and generative AI are transforming drug development, enhancing target accuracy and increasing the chances of clinical success.
Overview of AI Technologies in Pharma
AI technologies in pharma help find new drugs faster. They use machine learning, graphs, and big data to understand diseases. This helps predict how molecules will act as medicine.
AI looks at genes, pictures of cells, and information about chemicals. It finds patterns that humans might miss.
Tools like molecular simulations and graph neural networks play a big part. They make models of drug reactions without testing them in real life first. This saves time and money. AI can also design drugs from scratch and choose the best ones for more study.
These technologies are changing how we discover medicines.
Impact on the Drug Development Lifecycle
As AI technologies make their mark in pharma, their impact on the drug development lifecycle becomes clear. Artificial intelligence shortens timelines and cuts costs in drug development.
This is a big change for the industry. For example, by March 2022, Boston Consulting Group found over 150 small-molecule drugs were being discovered with an AI-first approach. Also, more than 15 of these drugs had started clinical trials.
AI improves how we find and manage diseases and run clinical trials. It makes identifying targets for new drugs faster and leads to better lead compound optimization. AI also plays a big role in designing new drugs and finding new uses for existing ones.
Plus, it helps make clinical trials more efficient. This means getting new treatments to patients quicker and at lower costs.
Key Applications of AI in Drug Discovery
AI plays a crucial role in identifying targets for drug development and speeding up the screening and optimization of compounds. It also aids in the pre-clinical and clinical development stages, as well as in FDA approval and post-market analysis.
Target Identification
Artificial intelligence helps find new targets for cancer treatment. It looks at how cells interact in the body. There are about 3,000 proteins that could be used to treat diseases out of 20,000 in our bodies.
AI can pick these out by studying our genes and how we change at the molecular level.
AI uses data to understand diseases better. It finds patterns in how genes work and how they relate to diseases like cancer. This makes it easier to find where to attack the disease.
AI turns complex data into clear targets for drugs, speeding up the discovery of treatments.
Virtual Screening and Optimization of Compounds
Now, let’s shift our focus from identifying potential drug targets to the next stage, which is the virtual screening and optimization of compounds. Virtual Screening (VS) makes use of computer-based methods to efficiently search through millions of compounds for potential drugs.
Machine Learning (ML) techniques play a crucial role in virtual screening by identifying promising drug leads using trained models.
In particular, Structure-Based Virtual Screening (SBVS) concentrates on the structures of ligands and targets, while Ligand-Based Screening (LBVS) relies on the chemical properties of known active compounds.
Key ML techniques involved in drug discovery include Naïve Bayes, k-Nearest Neighbors, Support Vector Machines, Random Forests, and Artificial Neural Networks. These advanced technologies significantly enhance the efficiency and speed at which potential drug candidates can be identified for further development.
Pre-clinical and Clinical Development
In February 2022, Insilico Medicine commenced Phase I clinical trials for the first AI-derived molecule identified based on a new target. This represents a significant milestone in drug development driven by AI, demonstrating its potential to expedite the process and bring innovative treatments to patients more rapidly.
Moreover, when considering cancer drugs specifically, it’s important to note that a remarkable 97% fail during clinical trials. This emphasizes the urgency and significance of leveraging advanced technologies such as AI to streamline pre-clinical and clinical development efforts.
Another pivotal example is AbSci’s accomplishment in January 2023, creating and validating de novo antibodies using generative AI. These instances highlight how AI is reshaping drug discovery processes by identifying novel targets and optimizing compounds more rapidly than traditional methods, addressing critical challenges related to time and cost efficiency within the drug development cycle.
FDA Approval and Post-market Analysis
AI is not just for drug discovery; it’s playing a role in the FDA approval process and post-market safety surveillance. In February 2023, the FDA granted its first Orphan Drug Designation to an AI-discovered drug, with Insilico Medicine planning a global Phase II trial early in 2023.
The FDA has received around 300 submissions referencing AI since 2016, showing the increasing integration of AI and machine learning into various stages of drug development, including post-market safety surveillance.
Furthermore, the FDA is developing a flexible, risk-based regulatory framework to promote AI innovation while ensuring patient safety.
Advanced AI Techniques Transforming Drug Discovery
Advanced AI techniques are revolutionizing drug discovery, from deep learning models for molecule generation to structure-based drug design and graph neural networks, unlocking new possibilities in accelerating pharmaceutical innovation with AI.
For more details on how these advancements are shaping the future of drug development, read our blog!
Deep Learning Models for Molecule Generation
Deep learning libraries like DeepChem and DeepAffinity contribute to drug discovery. They use AI and deep generative models, transforming medicinal applications. AlphaFold2 succeeded in predicting 3D protein structures in CASP14.
Graph neural networks (GNNs) aid molecule generation in drug discovery.
Structure-based Drug Design
AI is revolutionizing drug discovery, particularly in structure-based drug design. AlphaFold2, an AI-powered tool, greatly improves the prediction of crucial protein structures for this process.
Generative models are transforming approaches within the framework of structure-based drug design. Methods such as persistent homology enhance forecasts of binding affinities in protein-ligand complexes.
Molecular docking offers insights into drug-target interactions, assisting in structure-based design.
The future looks bright as AI continues to transform this field by advancing predictive analytics for personalized medicine and genomic research. Collaborative partnerships and ecosystems will further drive innovation in this constantly evolving field, ensuring more efficient and personalized drug development to enhance patient outcomes.
Next – Graph Neural Networks
Graph Neural Networks
Graph neural networks (GNNs) are advanced AI techniques transforming drug discovery. They model molecular systems and biomedical data relationships using graphs. For example, AbbVie is collaborating with Intel to use Federated Graph Neural Networks for training models while maintaining data privacy and intellectual property.
Initial experiments demonstrated the feasibility of GNN training in inter-graph settings within Federated Learning environments using PyTorch Geometric (PyG) and OpenFL. Promising results from experiments suggest that Federated Learning can effectively support GNN training in drug discovery applications, showcasing their potential to accelerate pharmaceutical research.
Moving on to “Data Representation and Analysis”…
Data Representation and Analysis
Transitioning from the realm of Graph Neural Networks to Data Representation and Analysis, advanced AI techniques play a pivotal role in transforming drug discovery. AI has the capability to model molecular systems and establish biomedical data relationships by utilizing graphs.
Machine-interpretable molecular representations elevate efficiency in drug discovery scenarios. Recent progress encompasses open-source tools, AI-compatible datasets, deep learning platforms for drug design, large language models (LLMs), and diffusion models as recommended strategies for data representation in AI drug development.
These advanced methodologies streamline the analysis of biomedical data and enhance the representation of intricate molecular structures crucial for formulating more potent drugs. Through graph-based data representation, artificial intelligence significantly contributes to bolstering the effectiveness and pace of drug discovery processes.
Challenges and Opportunities
Addressing challenges in data privacy and security while harnessing the opportunities presented by AI for drug discovery is crucial. Successfully dealing with data quality and quantity issues necessitates the integration of AI with traditional research methods to maximize its potential impact.
Navigating Data Privacy and Security
In the realm of drug discovery, addressing data privacy and security is crucial. Ethical considerations, regulatory frameworks, and patient data management are paramount. AI can help detect biases in real-world data that may affect patient outcomes.
Ensuring compliance with confidentiality measures and information security is essential to address ethical and regulatory challenges effectively.
Highlighting data governance, implementing security safeguards, and complying with regulations are crucial. It’s important to establish clear guidelines for using AI in drug discovery while focusing on protecting patient confidentiality within the constantly changing regulatory landscape.
Overcoming Data Quality and Quantity Issues
Data quality and quantity are crucial in AI-based drug discovery. The challenge lies in data scarcity, emphasizing the need for robust datasets for effective model training. Approaches like Transfer Learning, Active Learning, and Data Augmentation can handle low data scenarios effectively.
Moreover, data synthesis and federated learning serve as supplementary methods to address the issue of data quality and volume directly.
Effective handling is essential to ensure integrity, accuracy, completeness, validation, curation, governance, acquisition, enrichment normalization, and reliability in drug development influenced by AI technologies.
Integrating AI with Traditional Research Methods
Incorporating AI with traditional research methods presents promising opportunities for medical innovation and drug development. This fusion can lead to reduced research costs and increased success rates.
Particularly in addressing chronic diseases like diabetes and cancer, AI could help pioneer new advancements. Furthermore, the collaboration between AI and traditional plant-derived medicines offers an intriguing path for innovative therapeutic solutions.
It’s crucial to emphasize a strong framework for AI-assisted drug discovery, which highlights the necessity for ongoing enhancement in AI technologies to reveal the potential of combining traditional research methodologies with artificial intelligence.
Case Studies: AI Success Stories in Pharma
AI has led to major breakthroughs in Oncology Drug Discovery, Neurodegenerative Disease Research, and collaborative projects between AI startups and big pharma. Read more about these success stories!
AI in Oncology Drug Discovery
Artificial intelligence (AI) has significantly enhanced the efficiency and accuracy of oncology drug discovery. AI aids in identifying new drug targets for cancer treatments, improving the precision of predictions for drug candidates’ efficacy and toxicity in oncology.
For example, DeepMind’s AlphaFold plays a crucial role in influencing protein structure prediction concerning oncology. Machine learning algorithms also assist in forecasting the effectiveness of potential drugs for treating cancer, thus expediting the development process while upholding high accuracy levels.
The use of AI in oncology research has resulted in advancements that have positively influenced the speed and accuracy of drug discovery processes specifically tailored for cancer treatment.
These advancements have led to improved efficiency in predicting potential drug toxicity and enhancements in protein structure predictions relevant to oncology treatments.
AI-Powered Neurodegenerative Disease Research
AI is changing the game in finding treatments for neurodegenerative diseases. Companies like Verge Genomics are using AI to predict how drugs will affect patients with Alzheimer’s and Parkinson’s.
These simulations help optimize clinical trial designs, making it easier to recruit patients for studies on these conditions. By integrating AI, success rates in treating neurodegenerative diseases are expected to improve significantly.
Collaborative Projects Between AI Startups and Big Pharma
AI startups and big pharma are teaming up for collaborative projects to advance drug discovery. For example, the Novartis and Microsoft alliance uses AI to innovate drug development processes, streamlining operations.
These partnerships enable the exploration of AI’s potential in predicting drug-target interactions and optimizing drug repurposing strategies. They also promote better communication across various sectors involved in drug development, ultimately enhancing the industry’s innovation.
Collaborative ecosystems play a crucial role in addressing challenges related to data acquisition and analysis within the pharmaceutical realm. Such partnerships leverage AI technologies to navigate complexities in pharmaceuticals, offering customized solutions for predictive modeling and improving drug development outcomes.
Future Directions and Innovations
Future Directions and Innovations in drug discovery involve using predictive analytics for personalized medicine, integrating AI in genomic and biomedical research, and forming partnerships and collaborative ecosystems to drive advancements in the pharmaceutical industry.
These innovations strive to propel the field forward towards precision medicine and enhanced understanding of biological systems.
Predictive Analytics for Personalized Medicine
Predictive analytics and AI technology are revolutionizing personalized medicine. These advancements can positively impact patient care by using machine learning to customize treatments to each individual’s unique attributes.
They also aid clinicians in decision-making processes, particularly in analyzing genomic profiles for treatment forecasting and personalized care delivery. However, there are challenges, including the integration of health data from different sources, ensuring privacy and security in healthcare information systems, as well as addressing biases in medical data.
AI in Genomic and Biomedical Research
Transitioning from predictive analytics for personalized medicine to AI in genomic and biomedical research, it’s crucial to understand that AI is advancing the field, especially in small molecules, RNA, and antibodies.
Integration of large language models and diffusion models may help overcome challenges in AI pharmaceutical development. Recent advancements in knowledge graphs and graph neural networks are enhancing precision medicine initiatives.
Collaboration among academia, industry, and government is essential to address societal and ethical challenges in AI-driven drug development.
Partnerships and Collaborative Ecosystems
Collaborative efforts between biopharmaceutical companies and IT firms are crucial for advancing drug discovery with AI. These partnerships facilitate the integration of information technology, enhancing communication and collaboration in the pharmaceutical industry.
By leveraging AI’s capabilities, these collaborations enable predictive analytics for personalized medicine and optimize drug repurposing strategies. Furthermore, they play a vital role in overcoming data analysis challenges and addressing data privacy and security issues within the pharmaceutical realm.
These collaborative ecosystems pave the way for innovative approaches in partnerships, reinforcing advancements in drug discovery through artificial intelligence. The utilization of AI technologies fosters better communication across different sectors involved in drug development, emphasizing their importance in modernizing the process.
In addition, collaborative platforms aid in addressing challenges related to data acquisition and analysis by harnessing advanced tools and techniques that ultimately drive progress in this continually evolving field.
Leading into “Future Directions and Innovations” – Collaborations with Emerging Technologies
Conclusion
In closing, the use of Artificial Intelligence in drug discovery has revolutionized the biopharmaceutical industry. AI has significantly accelerated the process by predicting protein structures, designing novel drug molecules, and improving success rates.
This innovative approach holds promise for streamlining drug development and fostering a new era of pharmaceutical innovation. With continued advancements in AI technology, we can expect to see even greater strides in personalized medicine and improved patient outcomes.
Ultimately, AI is reshaping the future of drug discovery, marking an exciting chapter in pharmaceutical research.
FAQs
1. How does artificial intelligence speed up drug discovery?
Artificial intelligence speeds up drug discovery by analyzing vast amounts of data quickly. It identifies potential drug candidates and predicts their effectiveness.
2. What role does AI play in finding new medicines?
AI helps researchers find new medicines by simulating how drugs interact with biological systems. This reduces the time needed for testing and improves accuracy.
3. Can AI improve the safety of new drugs?
Yes, AI can enhance drug safety by predicting side effects early in the development process. This allows scientists to make better decisions about which compounds to pursue further.
4. Are there any challenges when using AI in drug discovery?
Challenges include data quality and integration issues. Researchers must ensure that the data used is accurate and relevant for effective results in discovering new drugs.